Geospatial intelligence (GEOINT) is the collection, analysis, and interpretation of data from Earth-based and space-based sources to provide insights into the physical features and activities on Earth. The Air Force is a major user of GEOINT, and it is increasingly using machine learning (ML) to improve its GEOINT capabilities. This article provides an in-depth analysis of the use of ML in Air Force GEOINT, including the benefits, challenges, and future of this technology.
Introduction:
Geospatial intelligence (GEOINT) refers to the exploitation and analysis of imagery and geospatial information to understand and visualize physical features and activities on Earth. With the emergence of advanced technologies like machine learning (ML) and artificial intelligence (AI), the integration of these technologies into GEOINT has revolutionized the way the Air Force operates. This article explores the use of geospatial intelligence and machine learning in the Air Force, highlighting key organizations and their locations.
Geospatial Intelligence in the Air Force
Geospatial intelligence provides the Air Force with critical information for a variety of military operations, including reconnaissance, surveillance, and target acquisition. It involves the collection, analysis, and dissemination of geospatial data to support decision-making processes. By utilizing geospatial information, the Air Force gains a comprehensive understanding of the operational environment, including terrain, infrastructure, and enemy activities.
Geospatial Intelligence and Machine Learning Integration
The integration of machine learning techniques into geospatial intelligence has significantly enhanced the Air Force’s analytical capabilities. Machine learning algorithms enable the automatic extraction of valuable insights from satellite imagery, aerial photographs, and other geospatial datasets. By training ML models on large volumes of labeled data, the Air Force can automate the process of identifying and classifying objects, structures, and activities on the ground. This integration significantly reduces the burden on human analysts and allows them to focus on higher-level decision-making tasks.
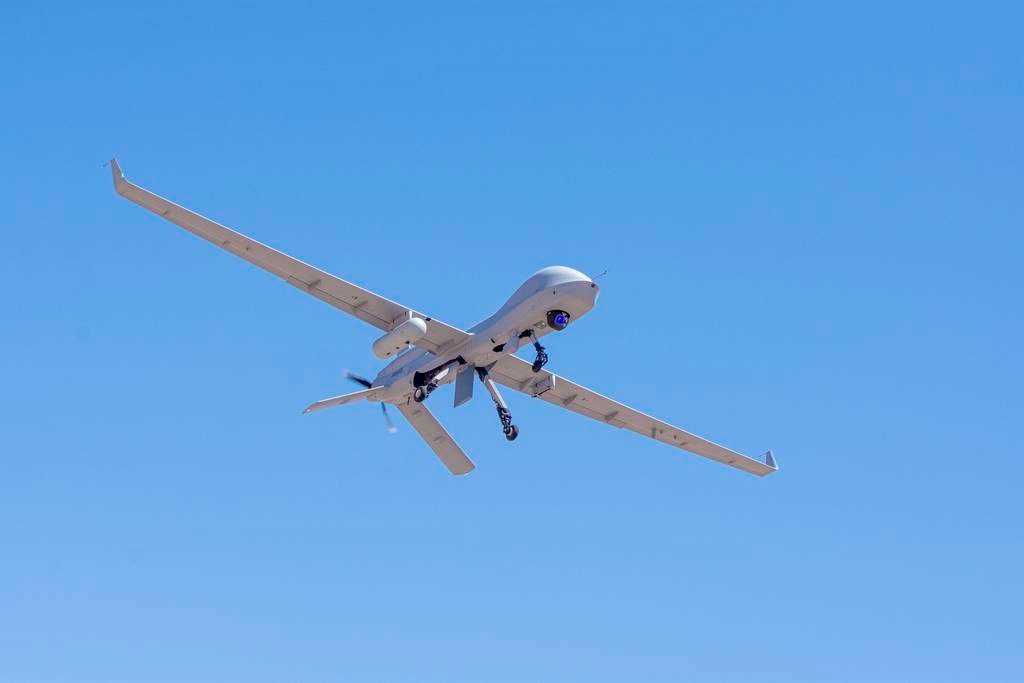
Organizations Utilizing Geospatial Intelligence and Machine Learning
1. National Geospatial-Intelligence Agency (NGA) – Springfield, Virginia:
The NGA is a key organization responsible for providing geospatial intelligence support to the U.S. Department of Defense (DoD) and other national security agencies. It leverages machine learning techniques to analyze vast amounts of satellite imagery and geospatial data, enabling the identification of potential threats, monitoring changes in enemy activities, and supporting mission planning. The NGA collaborates closely with the Air Force to provide timely and accurate geospatial intelligence for operational purposes.
2. Air Force Research Laboratory (AFRL) – Wright-Patterson Air Force Base, Ohio:
The AFRL conducts extensive research on geospatial intelligence and machine learning applications within the Air Force. It focuses on developing advanced algorithms and technologies to improve the collection, processing, and analysis of geospatial data. The integration of machine learning enables the AFRL to develop predictive models, anomaly detection systems, and automated object recognition tools, enhancing situational awareness and decision-making capabilities. The AFRL works closely with other research institutions and industry partners to advance the field of geospatial intelligence and machine learning.
3. 480th Intelligence, Surveillance, and Reconnaissance Wing – Joint Base Langley-Eustis, Virginia:
The 480th ISR Wing specializes in providing intelligence, surveillance, and reconnaissance support to combatant commanders and national agencies. It leverages geospatial intelligence and machine learning to process and analyze large volumes of sensor data, including radar, imagery, and signals intelligence. Machine learning algorithms enable the identification of patterns, anomalies, and emerging threats in real-time, supporting mission planning and targeting processes. The 480th ISR Wing plays a crucial role in gathering and analyzing geospatial information to support Air Force operations worldwide.
4. Air Combat Command (ACC) – Joint Base Langley-Eustis, Virginia: The ACC utilizes geospatial intelligence and machine learning techniques to enhance its operational capabilities. By integrating machine learning algorithms into its geospatial analysis workflows, the ACC can quickly identify and track hostile aircraft, detect changes in enemy activities, and assess potential target vulnerabilities.
Advancements in Geospatial Intelligence and Machine Learning
In recent years, advancements in geospatial intelligence and machine learning have opened up new possibilities for the Air Force. These advancements have improved the accuracy, efficiency, and timeliness of geospatial analysis, enabling the Air Force to make more informed decisions and respond rapidly to emerging threats. Let’s explore some of the key areas where geospatial intelligence and machine learning have made a significant impact.
Target Identification and Tracking
One of the primary applications of geospatial intelligence and machine learning in the Air Force is target identification and tracking. By analyzing satellite imagery and other geospatial data, machine learning algorithms can automatically detect and classify various targets, such as vehicles, buildings, and infrastructure. This capability allows the Air Force to quickly identify potential threats and track their movements, enhancing situational awareness and enabling timely response.
Predictive Analytics and Risk Assessment
Machine learning algorithms can analyze historical geospatial data to identify patterns and trends, enabling predictive analytics and risk assessment. By examining past events and activities, these algorithms can identify potential areas of interest or hotspots where future threats or incidents are likely to occur. This information allows the Air Force to allocate resources effectively and proactively plan operations to mitigate risks.
Mission Planning and Simulation
Geospatial intelligence combined with machine learning is invaluable in mission planning and simulation. By leveraging historical geospatial data and machine learning algorithms, the Air Force can simulate various scenarios and assess the potential outcomes of different courses of action. This capability enables the evaluation of mission feasibility, identification of optimal routes, and assessment of potential risks and obstacles. By leveraging geospatial intelligence and machine learning, the Air Force can plan and execute missions with greater precision and effectiveness.
Situational Awareness and Decision Support
Geospatial intelligence and machine learning play a crucial role in providing real-time situational awareness to commanders and decision-makers. By integrating geospatial data from multiple sources, such as satellites, drones, and ground-based sensors, with machine learning algorithms, the Air Force can monitor and analyze the operational environment in real-time. This enables rapid detection of changes, anomalies, or potential threats, allowing for timely decision-making and response.
Future Trends and Challenges
While geospatial intelligence and machine learning have transformed the Air Force’s capabilities, there are several future trends and challenges to consider.
Big Data and Data Integration
The volume and variety of geospatial data continue to increase exponentially, presenting challenges in terms of storage, processing, and integration. As the Air Force collects data from multiple sources, including satellites, sensors, and social media, effectively managing and integrating this data becomes crucial. Machine learning techniques for big data analytics and data fusion will play a vital role in extracting meaningful insights from this vast amount of information.
Ethical Considerations and Bias
As machine learning algorithms become more prevalent in geospatial intelligence, ethical considerations and potential biases need to be addressed. Bias in training data or algorithmic decision-making could lead to unfair outcomes or inaccurate predictions. The Air Force must ensure that machine learning algorithms are developed and deployed in a way that upholds ethical standards and avoids unintended biases.
Cybersecurity and Data Protection
As geospatial intelligence relies heavily on data from various sources, including classified information, ensuring cybersecurity and data protection is paramount. The Air Force must employ robust cybersecurity measures to safeguard geospatial data and prevent unauthorized access or manipulation. Machine learning models used in geospatial intelligence must also be protected from adversarial attacks to maintain the integrity and reliability of analytical outputs.
Conclusion:
Geospatial intelligence and machine learning have revolutionized the way the Air Force collects, analyzes, and utilizes geospatial data. Organizations such as the National Geospatial-Intelligence Agency (NGA), Air Force Research Laboratory (AFRL), 480th Intelligence, Surveillance, and Reconnaissance Wing, and Air Combat Command (ACC) are at the forefront of integrating geospatial intelligence and machine learning capabilities.
Through the integration of machine learning algorithms, the Air Force has enhanced its ability to identify and track targets, conduct predictive analytics, and support mission planning. Geospatial intelligence combined with machine learning provides the Air Force with improved situational awareness and decision support, enabling more effective and efficient operations.
Looking to the future, challenges such as managing big data, addressing ethical considerations, and ensuring cybersecurity and data protection will need to be addressed. The Air Force must continue to invest in research and development to advance geospatial intelligence and machine learning capabilities, while also upholding ethical standards and addressing potential biases.
In conclusion, the integration of geospatial intelligence and machine learning in the Air Force has significantly enhanced its operational capabilities. As technology continues to advance, geospatial intelligence and machine learning will play an increasingly critical role in supporting the Air Force’s mission to protect and defend the nation.
FAQs:-
Q1: What is geospatial intelligence (GEOINT) in the context of the Air Force?
A1: Geospatial intelligence (GEOINT) refers to the exploitation and analysis of imagery and geospatial information to understand and visualize physical features and activities on Earth. In the context of the Air Force, GEOINT provides critical information for various military operations, including reconnaissance, surveillance, and target acquisition.
Q2: How does machine learning integrate with geospatial intelligence in the Air Force?
A2: Machine learning (ML) algorithms are integrated into geospatial intelligence workflows to automate the analysis of vast amounts of geospatial data. ML models are trained on labeled data to identify and classify objects, structures, and activities on the ground, reducing the workload on human analysts and enhancing efficiency and accuracy in analyzing geospatial information.
Q3: Which organizations in the Air Force utilize geospatial intelligence and machine learning?
A3: Key organizations in the Air Force that utilize geospatial intelligence and machine learning include the National Geospatial-Intelligence Agency (NGA), Air Force Research Laboratory (AFRL), 480th Intelligence, Surveillance, and Reconnaissance Wing, and Air Combat Command (ACC). These organizations play a crucial role in advancing GEOINT and ML capabilities within the Air Force.
Q4: What are some applications of geospatial intelligence and machine learning in the Air Force?
A4: Geospatial intelligence and machine learning have various applications in the Air Force. These include target identification and tracking, predictive analytics and risk assessment, mission planning and simulation, and real-time situational awareness and decision support. ML algorithms enable the rapid and accurate analysis of geospatial data, providing valuable insights for operational planning and execution.
Q5: What are some future trends and challenges in geospatial intelligence and machine learning for the Air Force?
A5: Future trends and challenges in geospatial intelligence and machine learning for the Air Force include managing big data and data integration, addressing ethical considerations and potential biases, and ensuring cybersecurity and data protection. The increasing volume and variety of geospatial data, as well as the need for ethical and secure practices, will require ongoing research and development efforts.
Q6: How do geospatial intelligence and machine learning contribute to the Air Force’s mission?
A6: Geospatial intelligence and machine learning enhance the Air Force’s operational capabilities by providing timely and accurate information, improved situational awareness, and support for decision-making processes. By leveraging advanced analytics and automation, the Air Force can better understand the operational environment, track potential threats, optimize mission planning, and respond effectively to emerging challenges.
Q7: How do geospatial intelligence and machine learning benefit the Air Force compared to traditional methods?
A7: Geospatial intelligence and machine learning offer significant advantages over traditional methods in terms of speed, accuracy, and efficiency. ML algorithms can analyze vast amounts of geospatial data in real-time, automating tasks that would otherwise require extensive manual effort. This enables the Air Force to process information more quickly, make more informed decisions, and allocate resources more effectively.
Q8: Are there any ethical considerations in the use of geospatial intelligence and machine learning in the Air Force?
A8: Yes, the use of geospatial intelligence and machine learning in the Air Force raises ethical considerations. Ensuring unbiased data collection, addressing potential biases in algorithms, and protecting sensitive data and privacy are crucial aspects that must be carefully managed. The Air Force is committed to upholding ethical standards and ensuring responsible use of geospatial intelligence and machine learning technologies.